The Kryptonite of AI: Unveiling the Hidden Weaknesses of Artificial Intelligence
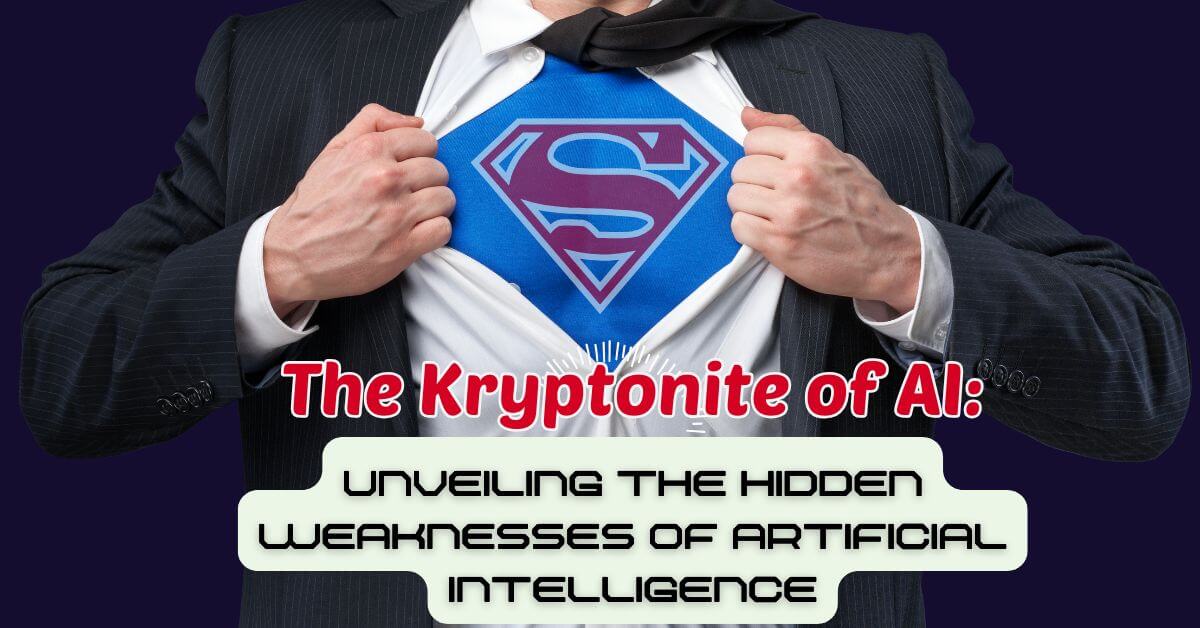
In the realm of technology, Artificial Intelligence stands as our modern-day Superman – powerful, fast, and seemingly unstoppable. Yet, just like the Man of Steel has his kryptonite, AI systems face their own set of fundamental limitations that remind us they’re far from invincible. Let’s dive into what makes these digital superheroes stumble.
The Superman Paradox: Understanding AI’s Current Powers
Today’s AI can perform impressive feats: beating world champions at complex games, generating art that sells for thousands of dollars, and even engaging in convincing conversations. Companies like OpenAI, DeepMind, and Anthropic have pushed the boundaries of what seemed possible just a few years ago. However, this surface-level excellence often masks deeper limitations.
What Makes AI Stumble: Core Limitations
Unlike Superman’s single weakness, AI faces multiple “kryptonite” challenges. Perhaps the most significant is common sense reasoning – that uniquely human ability to understand context and make intuitive decisions. While an AI can process billions of calculations per second, it might struggle with simple tasks that any child can handle, like understanding why you can’t put an elephant in a refrigerator.
Another crucial limitation lies in emotional intelligence. Despite advances in natural language processing, AI systems don’t truly understand or feel emotions – they pattern match based on training data. This creates a fundamental barrier in situations requiring genuine empathy or emotional nuance, particularly in healthcare or counseling scenarios.
The Human Edge: Tasks Where Humans Still Reign Supreme
Humans continue to outperform AI in several key areas. Creative problem-solving in novel situations remains distinctly human – while AI can combine existing ideas in new ways, it struggles with truly original thinking. Consider how a chef can improvise with unexpected ingredients, while AI cooking systems need precise recipes and parameters.
Understanding causation versus correlation also remains a human strength. AI systems excel at finding patterns but often can’t distinguish between meaningful relationships and coincidental correlations. This limitation becomes particularly evident in scientific research and medical diagnosis.
The Filipino Perspective: AI in a Relationship-Centered Culture
In the Philippines, where “bayanihan” spirit and close family ties reign supreme, AI faces unique cultural challenges. Take the concept of “pakikisama” – the Filipino value of smooth interpersonal relationships. While AI can translate “Kumusta ka?” literally, it misses the deep cultural context of genuine concern and relationship-building behind this simple greeting.
Consider how AI handles the nuanced communication in Filipino business settings. In traditional Filipino meetings, reading between the lines and understanding non-verbal cues is crucial. When someone says “bahala na,” it’s not just about leaving things to chance – it’s a complex cultural expression that AI struggles to grasp. Similarly, in customer service, where many Filipino BPO professionals excel, AI chatbots can’t replicate the naturally empathetic and patient approach that Filipino customer service representatives are known for worldwide.
The “utang na loob” (debt of gratitude) concept presents another fascinating challenge for AI. In Filipino society, this implicit system of reciprocal obligations helps maintain social harmony, but AI systems can’t understand these unwritten rules that govern many social and professional interactions in the Philippines. For instance, while AI can process transactions in online banking apps like GCash or Maya, it can’t understand the social implications of sending “pamasko” during Christmas or the delicate balance of gift-giving during fiestas.
Future-Proofing: Can AI Overcome Its Kryptonite?
The path forward isn’t about eliminating these limitations but rather understanding and working with them. Researchers are developing new approaches to make AI more robust and reliable. For instance, techniques like few-shot learning are helping AI systems perform better with limited data, while advances in embodied AI are improving common-sense reasoning.
Hardware limitations present another significant challenge. The energy consumption of large AI models has become a growing concern, with some estimates suggesting that training a single large language model can generate as much carbon dioxide as five cars over their lifetimes.
Looking Ahead: The Human-AI Partnership
The key to future success lies not in trying to eliminate AI’s kryptonite but in creating synergistic partnerships between human and artificial intelligence. By understanding these limitations, we can better design systems that complement human capabilities rather than attempt to replace them entirely.
Sources and Further Reading:
- “AI Index Report 2024” – Stanford University
- “The Limitations of Deep Learning” – Nature Machine Intelligence
- “Energy and Policy Considerations for Deep Learning in NLP” – ACL Proceedings
- “Common Sense Knowledge in Machine Learning” – MIT Technology Review
- Technical reports from OpenAI, DeepMind, and Google Research
Note for readers: This article was written in early 2024, and given the rapid pace of AI development, some limitations discussed may have seen progress since publication. Always refer to latest research for current state of AI capabilities.